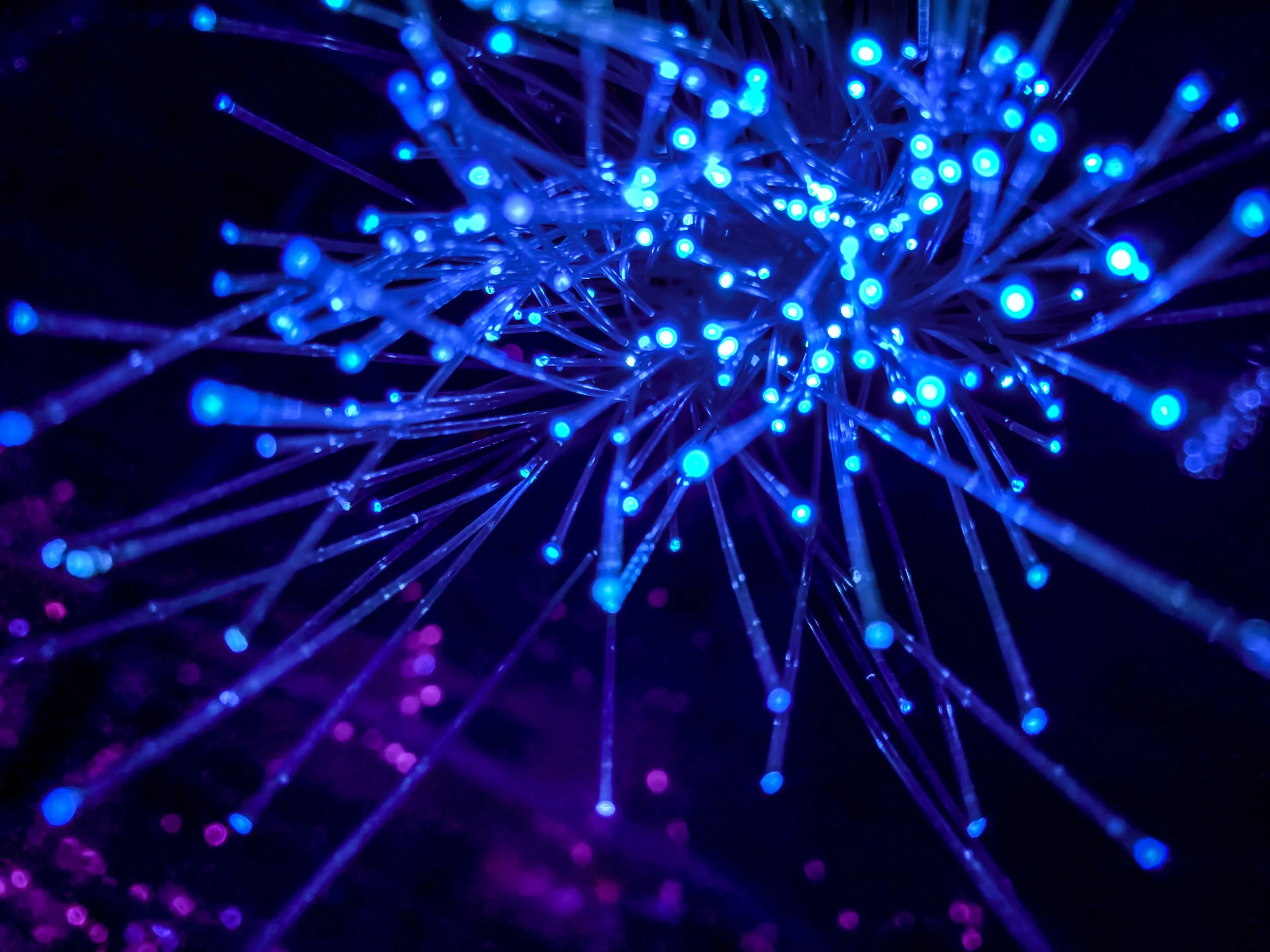
Integrating machine learning and genomics
We’re harnessing the power of artificial intelligence to develop predictive model for medication response in the clinic.
Image credit: JJ Ying
Understanding complex psychiatric disorders
The complexity of psychiatric disorders requires the application of advanced statistical methods to develop robust predictive models for antidepressant remission. Machine learning (ML) focuses on the prediction of unobserved or future outcomes, such as whether an individual with specific clinical characteristics will respond to a medication.
While machine learninf approaches are only slowly gaining attention in psychiatric research, is already widely applied and shows exceptional performance in oncology and radiogenomics. The success of machine learning may be attributed to the in-depth discussion of how to conduct rigorous, well-designed machine learning studies, as well as how to evaluate, validate, and report results.
pipeR: An Automated Pipeline for Predictive Modelling using Genome-wide Data (v.0.0.0.9070)
We have developed a systematic computational pipeline (R package pipeR) which integrates clinical and genome-wide data to build predictive models. Currently, using our pipeline, we are investigating various parameters including outcome complexity, genetic pruning threshold, genetic coding, sample balance, feature prioritization, feature selection and algorithm selection which may impact model performance.
Current Investigations
Currently, our goal is to understand and predict antidepressant remission in older adults with depression using demographic, clinical and genetic characteristics. To achieve these goals, we are (1) evaluating the usage of multiple polygenic risk scores (PRS) and single nucleotide polymorphisms (SNPs) to predict remission, and (2) characterizing metabolic pathways and protein-protein interaction networks associated with predictive, genetic traits. Here, we define predictive within the context of machine learning, as making a prediction for important clinical outcomes (e.g., remission).
Phase I
Evaluating the performance of predictive multiple PRS models to predict antidepressant treatment remission in late-life depression.
Phase II
Building SNP-based, machine learning models to predict antidepressant treatment remission in LLD and evaluating their predictive performance in comparison to the models using multiple PRS.
Phase III
Exploring the biological processes and molecular pathways associated with the most predictive genetic variables (i.e., SNPs) from our multiple PRS models
Did you know?
While machine learning approaches are only slowly gaining attention in psychiatric research, is already widely applied and shows exceptional performance in oncology and radiogenomics.
Selected Publications
GWAS-based machine learning approach to predict duloxetine response in major depressive disorder.
Maciukiewicz M, Marshe VS, Hauschild AC, Foster JA, Rotzinger S, Kennedy JL, Kennedy SH, Müller DJ, Geraci J. J Psychiatr Res. 2018 Apr;99:62-68.